The future of data management has arrived - Microsoft Fabric is…
As with any revolution, the evolution of data science brings great benefits along with new problems. Technology keeps progressing—introducing questions we’ve never had to ask. These ever-growing issues from data ownership to data privacy are hardly ever black and white, and the evolving solution often sets a precedent for our lives in the future. Machine learning’s popularity has skyrocketed in the last five years and become ubiquitous in our data world. And while the potential of machine learning is unquestionable, its application has created some ethical issues that have been overshadowed.
This makes sense. Not many people are at the decision level of data science to really worry about the nitty-gritty ethics involved. But its universal impact makes data ethics an important growing conversation as more and more companies’ mistakes come to light. Algorithmic bias has been a prominent topic in the news with powerhouse companies like Google and Amazon being named culprits. But this crime is most often unintentional.
Algorithmic bias is the phenomenon of algorithms systematically producing discriminatory outcomes. It may be puzzling to read at first because we are used to associating math with pure, hard logic. Can math really be biased? Algorithms are just functions of their data. This data has been created and curated by humans, so it can reflect and even magnify our biases in algorithmic outcomes. This can be dangerous when deciding the reality of individuals in the real world like in employment and criminal justice.
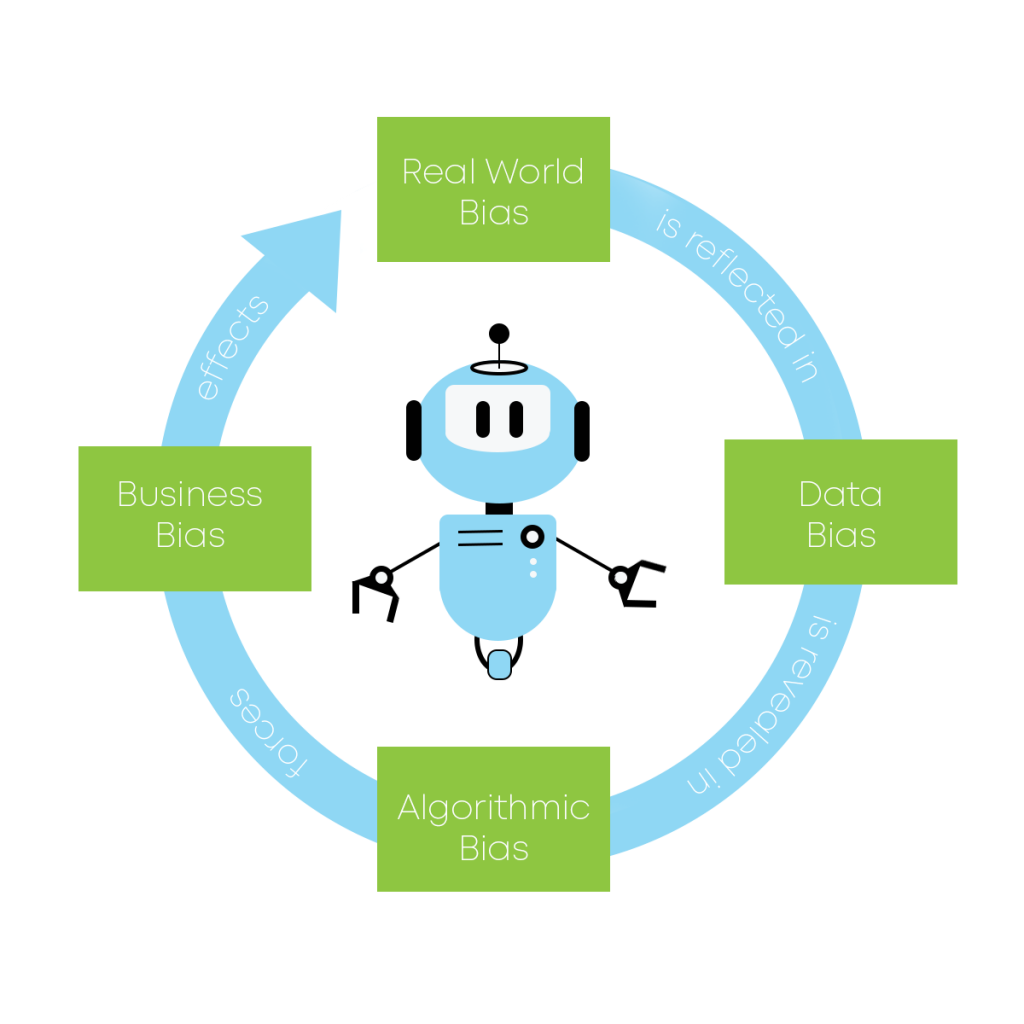
Algorithmic Bias Exists
Now we’ll look at a few significant instances where algorithmic bias has occurred. Increasingly, judges and parole officers consider a ‘risk score’ from a risk assessment algorithm when deciding a defendant’s likelihood of reoffending. This score can ultimately influence decisions on bailouts and sentencing. ProPublica launched an investigation to evaluate accuracy and bias of certain groups on one of the leading risk assessment algorithms COMPAS (Correctional Offender Management Profiling for Alternative Sanctions) by the commercial company Northpoint Incorporated. Their study found black defendants to be twice as likely as white defendants to be falsely identified as high risk. In contrast, white defendants were almost twice as likely to be falsely identified as low risk than black defendants. Another study on COMPAS by Darmouth Professor Hany Farid and his student Julia Dressel, show that racial discrimination still slipped into models that do not include race classes through proxy features like prior criminal charges.
Race is another tremendous issue concerning facial recognition systems. In 2018, MIT researchers published their study in the New York Times on racial bias found in facial recognition systems from big brands like Microsoft, IBM, and Amazon. Their findings: women of darker skin tones were the highest misidentified at an error rate of 21 to 35 percent while the maximum error rate for men of lighter skin tones was less than one percent. Alarmingly, the researchers reported that a ‘major U.S. technology company’ used a dataset that was 83 percent white and 77 percent male to train its software.
Gender discrimination can occur in similar fashion to race. Many companies have pushed to make their hiring process more efficient by the addition of automation, including recruitment algorithms in their decisions. Amazon’s AI recruitment tool was ultimately scrapped when they realized it disadvantaged women. The recruitment tool favored masculine words while penalizing for the usage of the word ‘women’ in extracurriculars. Algorithms can’t be smarter than their data. And in this case, the training data was 10 years of applicant’s resumes from the androcentric technology industry.
All of these algorithms arise from innovation and good intentions, these occurrences of bias were accidental. However, they all have real and impactful consequences.
Unintended Consequences
Since algorithms are so deeply integrated into our lives, they now shape our world. Their bias can potentially lead to heavy societal consequences. Algorithmic methods have the propensity to learn the current state of the world, referred to as ossification. This can make the world harder to change. AI can only learn from the data we provide, and ossification may unconsciously promote society’s unfair biases as demonstrated in the Amazon’s recruitment use case.
From music to news articles, recommendation algorithms by design feed us what we want—trapping us in our own perspective. The resulting echo chamber can create a feedback loop where we only encounter information that reinforces our current view. AI is powerful but also malleable and influential. We must take caution to not propel the future toward a “boy’s club” or an insular world view due to ossification and echo chambers.
How Zirous Can Help
An inherent advantage of algorithms is the ability to quantify their bias straightforwardly and, within a certain scope, prove fairness. However, in the common move-fast-and-break-things mentality of the tech world, ethical practice conversations may not be prioritized. At Zirous, we make explicit efforts to help you prevent bias by watching the following two common pitfalls:
- Big data does not solve everything. A common misconception in the data age is that more means better. This isn’t necessarily true. Large datasets may decrease margin of error but have the potential to magnify underlying biases. We make no assumptions and scrutinize your data in thorough exploratory data analysis, laying the foundation for your data-driven business. To begin developing your Unified Analytics Strategy, Zirous offers our Catalyst program.
- Protected classes need special attention. Examples of sensitive classes include, but are not limited to: gender, race, socioeconomic level, and location. Zirous is especially diligent with these classes because they can be hidden in proxy features or misrepresented in data.
Overall, recognizing that bias exists and actively looking for it is a major first step in preventing it. It’s important to remember how easily algorithms can be swayed. The data could be biased or the system could be biased, leading to unintentional results of “black box” algorithms. We should note that not all machine learning situations carry significant societal impact from bias. But it is the instances affecting individuals that we should be particularly heedful of ethical practices.
All of this may seem intimidating, but we are here to do all the heavy lifting. Machine learning is helping businesses achieve actionable insights, drive decisions, and develop revolutionary products. Zirous can help your business utilize machine learning with a Spark engagement. Contact us to learn more.
This Post Has 0 Comments